期刊全称 | Artificial Neural Networks and Machine Learning -- ICANN 2012 | 期刊简称 | 22nd International C | 影响因子2023 | Alessandro E. P. Villa,Włodzisław Duch,Günther Pal | 视频video | | 发行地址 | Uo to date results Fast track conference proceedings.State-of-the-Art research | 学科分类 | Lecture Notes in Computer Science | 图书封面 | 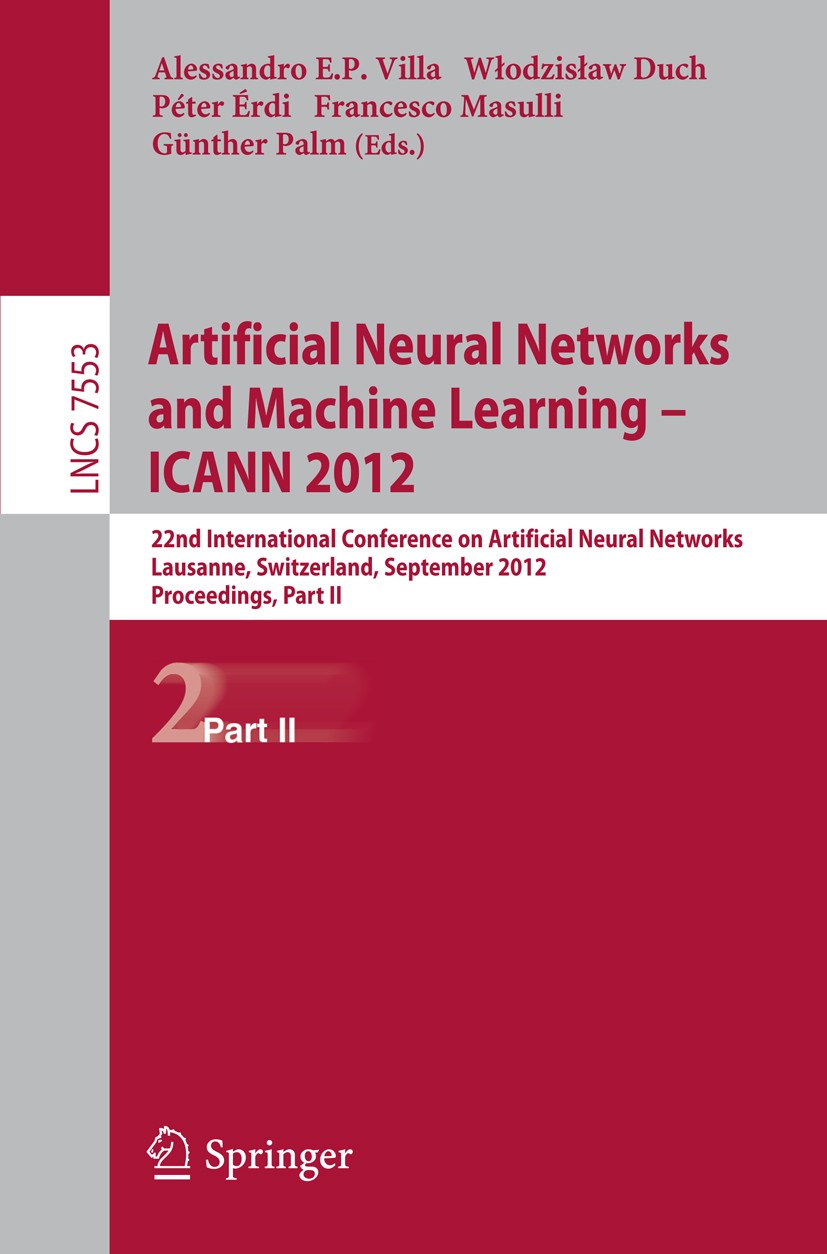 | 影响因子 | The two-volume set LNCS 7552 + 7553 constitutes the proceedings of the 22nd International Conference on Artificial Neural Networks, ICANN 2012, held in Lausanne, Switzerland, in September 2012. The 162 papers included in the proceedings were carefully reviewed and selected from 247 submissions. They are organized in topical sections named: theoretical neural computation; information and optimization; from neurons to neuromorphism; spiking dynamics; from single neurons to networks; complex firing patterns; movement and motion; from sensation to perception; object and face recognition; reinforcement learning; bayesian and echo state networks; recurrent neural networks and reservoir computing; coding architectures; interacting with the brain; swarm intelligence and decision-making; mulitlayer perceptrons and kernel networks; training and learning; inference and recognition; support vector machines; self-organizing maps and clustering; clustering, mining and exploratory analysis; bioinformatics; and time weries and forecasting. | Pindex | Conference proceedings 2012 |
The information of publication is updating
|
|