期刊全称 | Artificial Intelligence and Machine Learning | 期刊简称 | 32nd Benelux Confere | 影响因子2023 | Mitra Baratchi,Lu Cao,Frank W. Takes | 视频video | | 学科分类 | Communications in Computer and Information Science | 图书封面 | 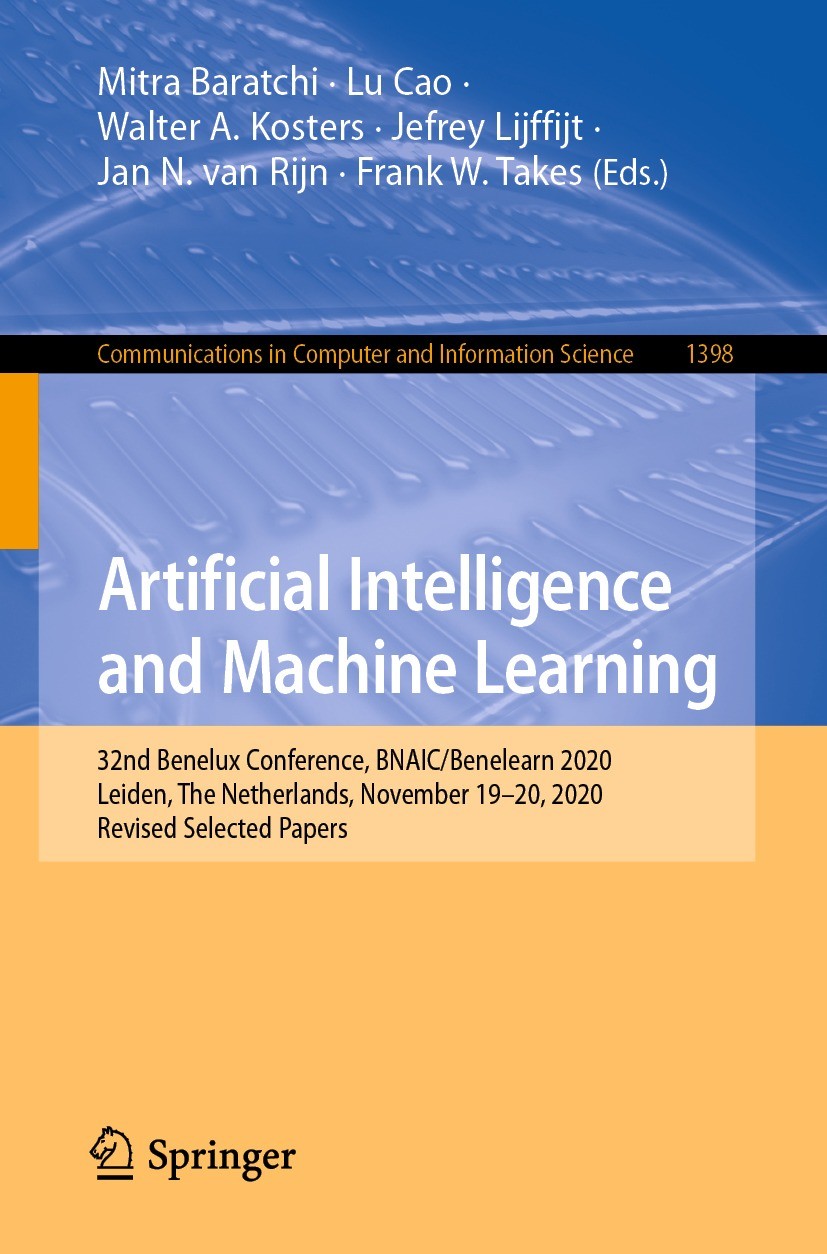 | 影响因子 | This book contains a selection of the best papers of the 32nd Benelux Conference on Artificial Intelligence, BNAIC/Benelearn 2020, held in Leiden, The Netherlands, in November 2020. Due to the COVID-19 pandemic the conference was held online. .The 12 papers presented in this volume were carefully reviewed and selected from 41 regular submissions. They address various aspects of artificial intelligence such as natural language processing, agent technology, game theory, problem solving, machine learning, human-agent interaction, AI and education, and data analysis..The chapter 11 is published open access under a CC BY license (Creative Commons Attribution 4.0 International License) .Chapter “Gaining Insight into Determinants of Physical Activity Using Bayesian Network Learning” is available open access under a Creative Commons Attribution 4.0 International License via link.springer.com.. . | Pindex | Conference proceedings 2021 |
The information of publication is updating
|
|