期刊全称 | Advances in Self-Organizing Maps, Learning Vector Quantization, Clustering and Data Visualization | 期刊简称 | Proceedings of the 1 | 影响因子2023 | Alfredo Vellido,Karina Gibert,José David Martín Gu | 视频video | | 发行地址 | Covers the latest theoretical developments in Self-Organizing Maps, Learning Vector Quantization, Clustering and Data Visualization.Presents computational aspects and applications for data mining and | 学科分类 | Advances in Intelligent Systems and Computing | 图书封面 | 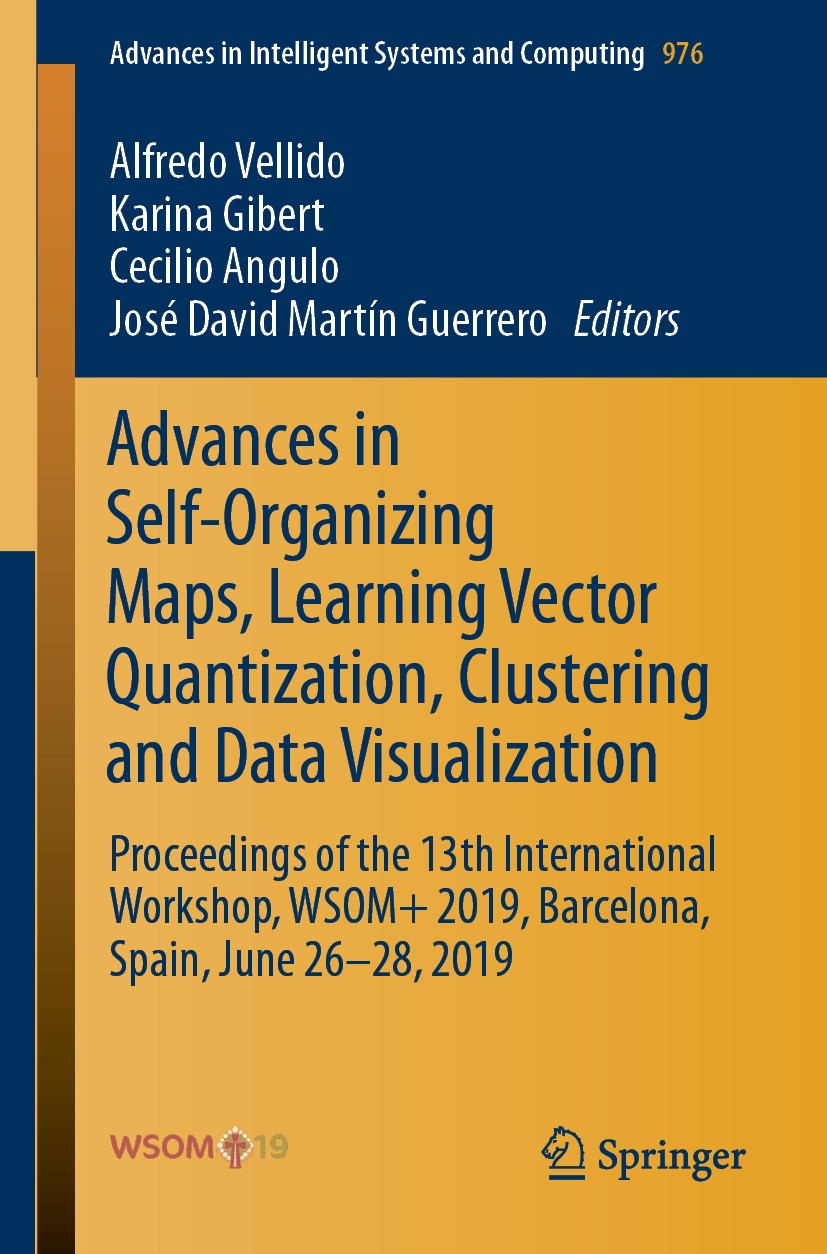 | 影响因子 | .This book gathers papers presented at the 13th International Workshop on Self-Organizing Maps, Learning Vector Quantization, Clustering and Data Visualization (WSOM+), which was held in Barcelona, Spain, from the 26th to the 28th of June 2019. Since being founded in 1997, the conference has showcased the state of the art in unsupervised machine learning methods related to the successful and widely used self-organizing map (SOM) method, and extending its scope to clustering and data visualization. In this installment of the AISC series, the reader will find theoretical research on SOM, LVQ and related methods, as well as numerous applications to problems in fields ranging from business and engineering to the life sciences. Given the scope of its coverage, the book will be of interest to machine learning researchers and practitioners in general and, more specifically, to those looking for the latest developments in unsupervised learning and data visualization.. | Pindex | Conference proceedings 2020 |
The information of publication is updating
书目名称Advances in Self-Organizing Maps, Learning Vector Quantization, Clustering and Data Visualization影响因子(影响力) 
书目名称Advances in Self-Organizing Maps, Learning Vector Quantization, Clustering and Data Visualization影响因子(影响力)学科排名 
书目名称Advances in Self-Organizing Maps, Learning Vector Quantization, Clustering and Data Visualization网络公开度 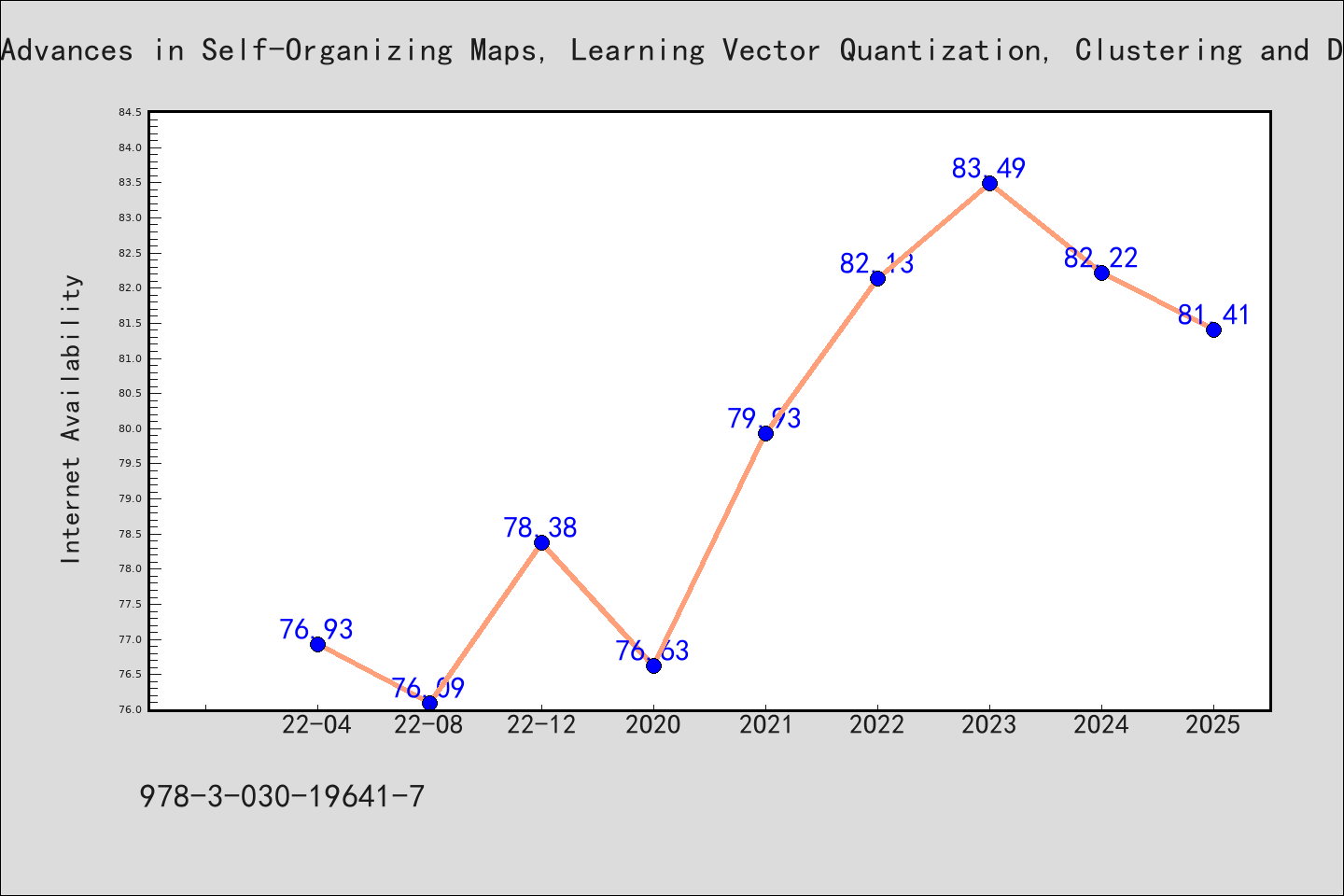
书目名称Advances in Self-Organizing Maps, Learning Vector Quantization, Clustering and Data Visualization网络公开度学科排名 
书目名称Advances in Self-Organizing Maps, Learning Vector Quantization, Clustering and Data Visualization被引频次 
书目名称Advances in Self-Organizing Maps, Learning Vector Quantization, Clustering and Data Visualization被引频次学科排名 
书目名称Advances in Self-Organizing Maps, Learning Vector Quantization, Clustering and Data Visualization年度引用 
书目名称Advances in Self-Organizing Maps, Learning Vector Quantization, Clustering and Data Visualization年度引用学科排名 
书目名称Advances in Self-Organizing Maps, Learning Vector Quantization, Clustering and Data Visualization读者反馈 
书目名称Advances in Self-Organizing Maps, Learning Vector Quantization, Clustering and Data Visualization读者反馈学科排名 
|
|
|