期刊全称 | Advances in Self-Organizing Maps, Learning Vector Quantization, Clustering and Data Visualization | 期刊简称 | Dedicated to the Mem | 影响因子2023 | Jan Faigl,Madalina Olteanu,Jan Drchal | 视频video | | 发行地址 | Provides recent research in self-organizing maps, learning vector quantization, clustering, and data visualization.Presents computational aspects and applications for data mining and visualization.Con | 学科分类 | Lecture Notes in Networks and Systems | 图书封面 | 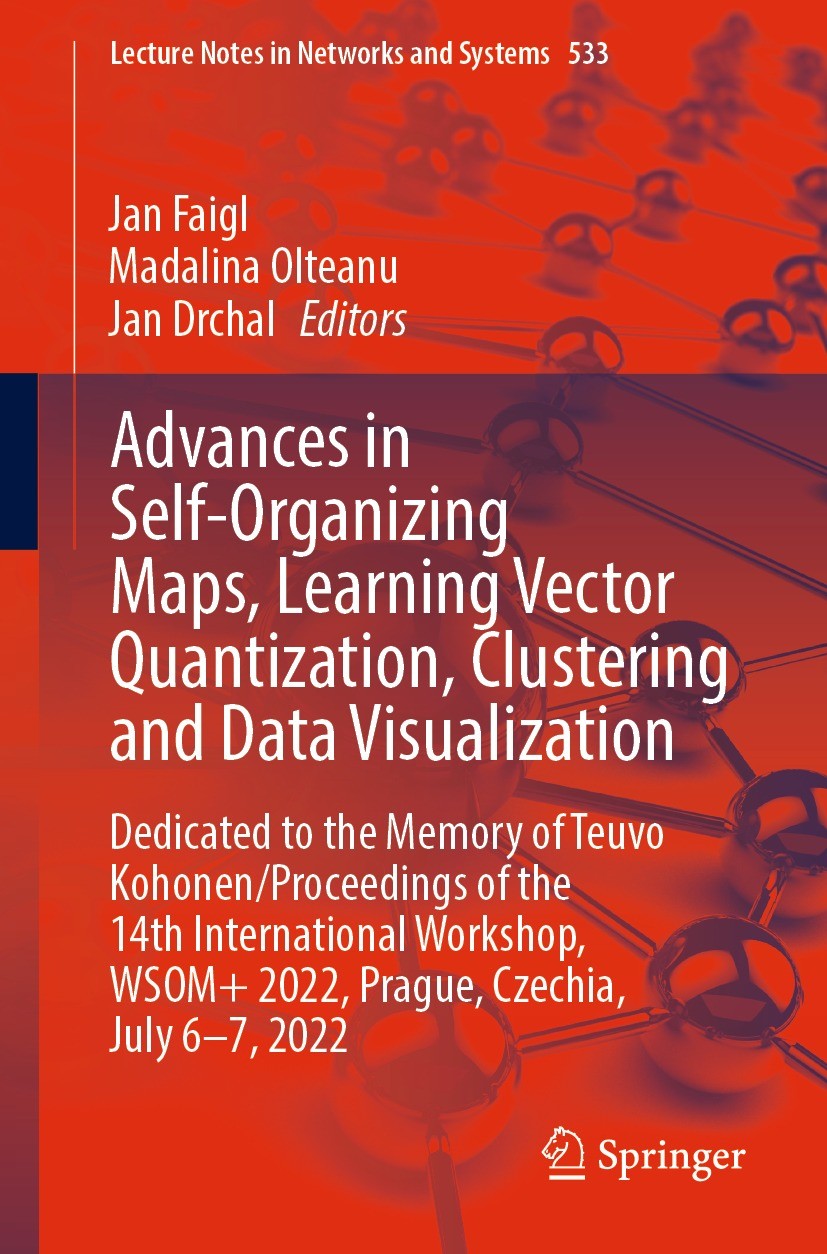 | 影响因子 | .In this collection, the reader can find recent advancements in self-organizing maps (SOMs) and learning vector quantization (LVQ), including progressive ideas on exploiting features of parallel computing. The collection is balanced in presenting novel theoretical contributions with applied results in traditional fields of SOMs, such as visualization problems and data analysis. Besides, the collection further includes less traditional deployments in trajectory clustering and recent results on exploiting quantum computation. The presented book is worth interest to data analysis and machine learning researchers and practitioners, specifically those interested in being updated with current developments in unsupervised learning, data visualization, and self-organization.. | Pindex | Conference proceedings 2022 |
The information of publication is updating
书目名称Advances in Self-Organizing Maps, Learning Vector Quantization, Clustering and Data Visualization影响因子(影响力) 
书目名称Advances in Self-Organizing Maps, Learning Vector Quantization, Clustering and Data Visualization影响因子(影响力)学科排名 
书目名称Advances in Self-Organizing Maps, Learning Vector Quantization, Clustering and Data Visualization网络公开度 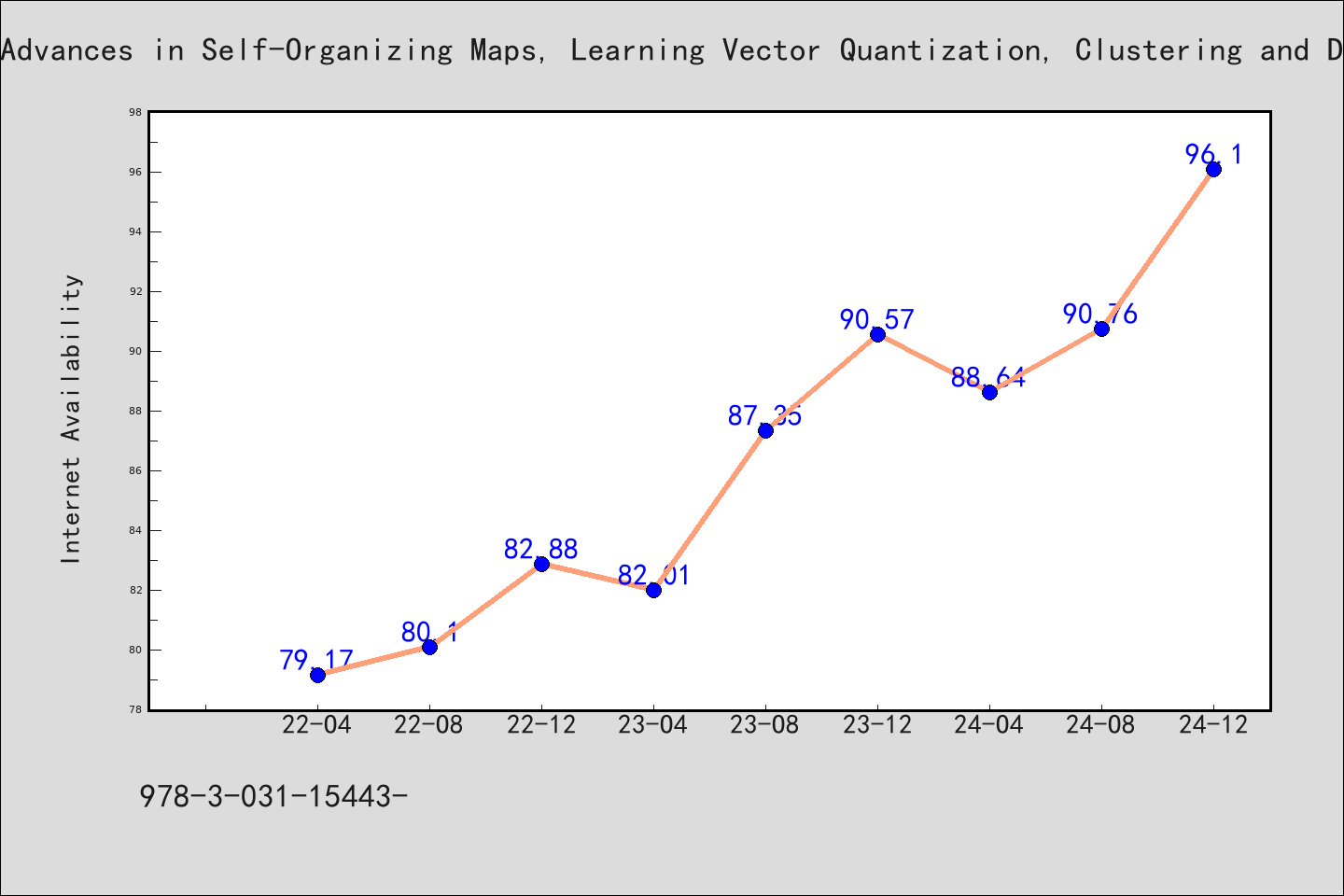
书目名称Advances in Self-Organizing Maps, Learning Vector Quantization, Clustering and Data Visualization网络公开度学科排名 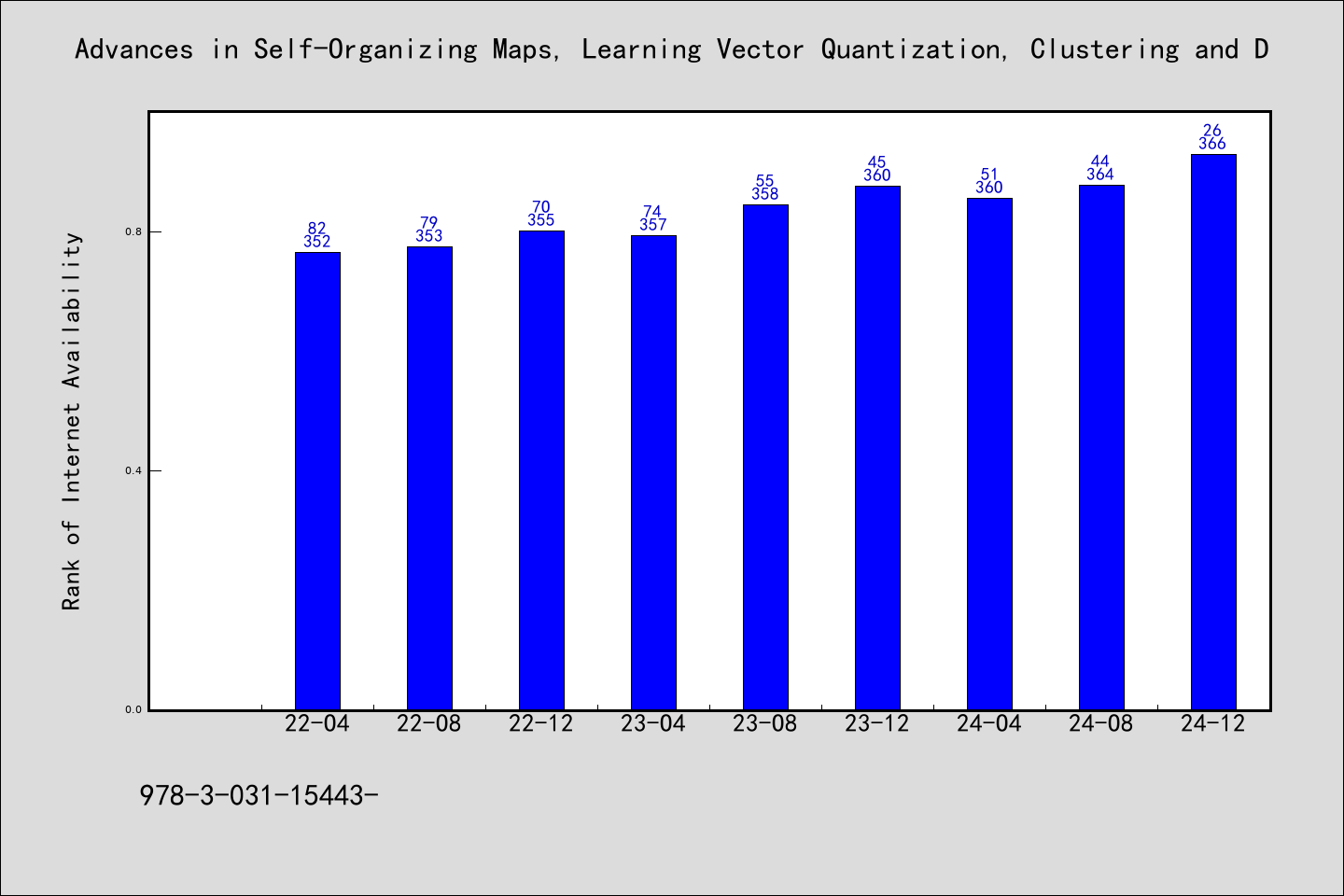
书目名称Advances in Self-Organizing Maps, Learning Vector Quantization, Clustering and Data Visualization被引频次 
书目名称Advances in Self-Organizing Maps, Learning Vector Quantization, Clustering and Data Visualization被引频次学科排名 
书目名称Advances in Self-Organizing Maps, Learning Vector Quantization, Clustering and Data Visualization年度引用 
书目名称Advances in Self-Organizing Maps, Learning Vector Quantization, Clustering and Data Visualization年度引用学科排名 
书目名称Advances in Self-Organizing Maps, Learning Vector Quantization, Clustering and Data Visualization读者反馈 
书目名称Advances in Self-Organizing Maps, Learning Vector Quantization, Clustering and Data Visualization读者反馈学科排名 
|
|
|