期刊全称 | Advances in Intelligent Data Analysis XXI | 期刊简称 | 21st International S | 影响因子2023 | Bruno Crémilleux,Sibylle Hess,Siegfried Nijssen | 视频video | | 学科分类 | Lecture Notes in Computer Science | 图书封面 | 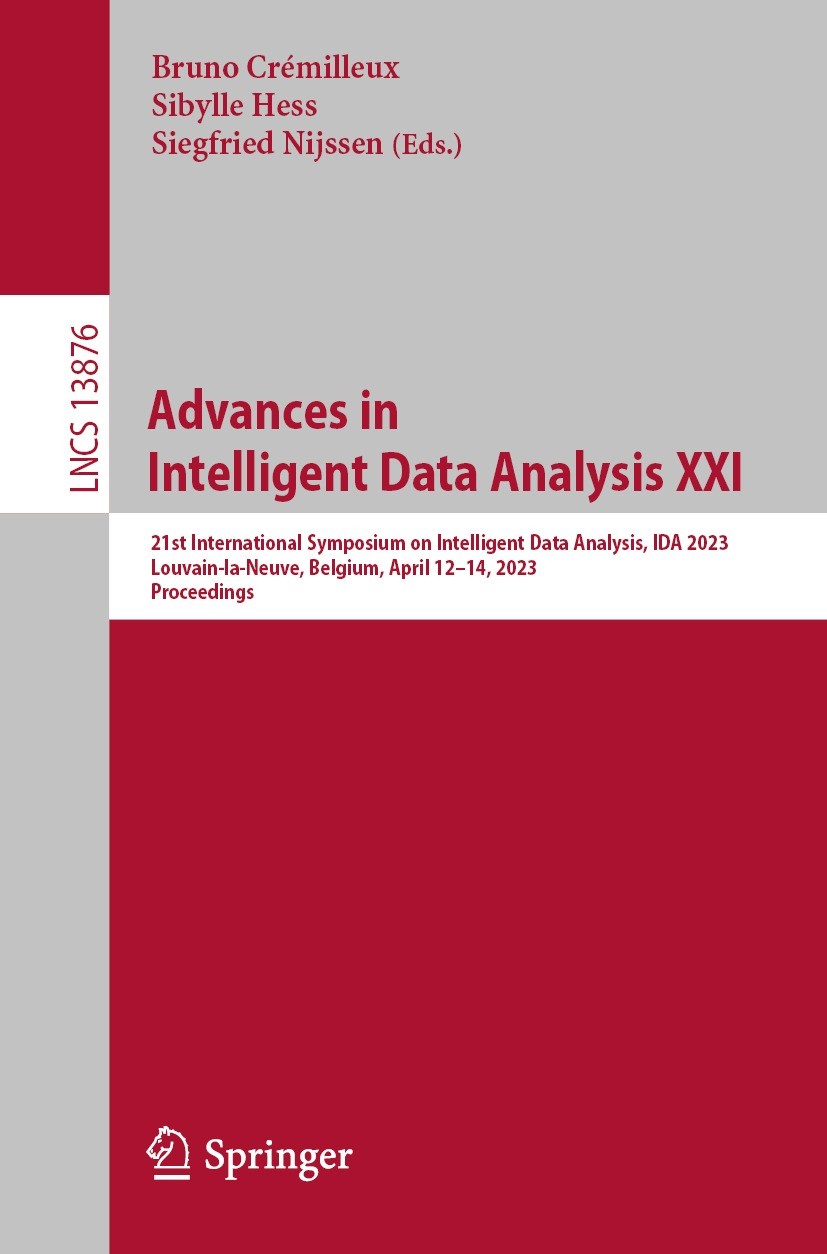 | 影响因子 | .This book constitutes the proceedings of the 21st International Symposium on Intelligent Data Analysis, IDA 2022, which was held in Louvain-la-Neuve, Belgium, during April 12-14, 2023...The 38 papers included in this book were carefully reviewed and selected from 91 submissions. IDA is an international symposium presenting advances in the intelligent analysis of data. Distinguishing characteristics of IDA are its focus on novel, inspiring ideas, its focus on research, and its relatively small scale. . | Pindex | Conference proceedings 2023 |
The information of publication is updating
|
|